Data Privacy
Data privacy is concerned with ensuring the appropriateness of information flows in our digital world. We research various areas of data privacy, ranging from theory and fundamentals of privacy notions such as Differential Privacy (DP), its application to modern data processing methods such as machine learning, to practical applications of biometric data (e.g. in authentication) and their anonymization (e.g. in video recordings).
The following sections provide an overview of the different topics that we research at the chair.
Evaluating and Designing Anonymization Methods for Video
Videos are captured ubiquitously in everyday life. This poses a risk to privacy, as biometric recognition methods can successfully infer identities and sensitive attributes of the persons captured in the video material. We aim to protect the privacy of people in video material, while still preserving the utility of the collected data.
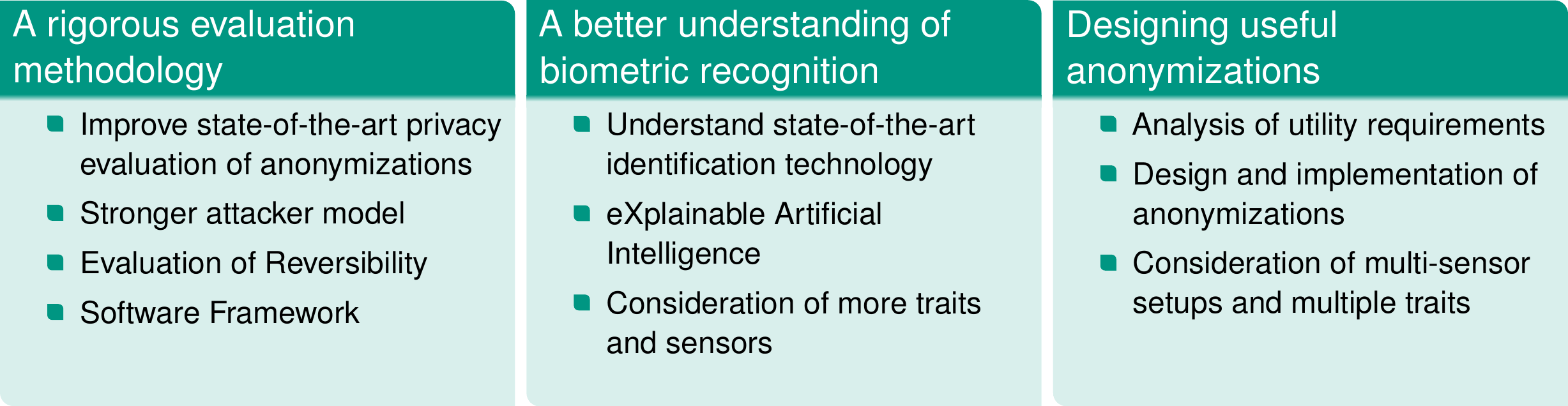
Use cases
- Applications like smart cities, autonomous driving and medicine can profit from collected data
- Allow these domains to use available data while protecting the privacy of individuals in the videos
Methods
- Machine learning based attackers for evaluation
- Developing new anonymization methods using machine learning
- User studies to validate experimental results and to create data sets
Future
- Consideration of more biometric traits and their combination
- Better evaluate the utility of anonymizations
- Anonymizations that provide the utility required for more applications
Protecting Human Trajectory Data
Patricia Guerra-Balboa: Local DP Trajectory Anonymization
Àlex Miranda-Pascual: Enhancing Utility in DP Trajectory Protection
Trajectory data analysis can improve our daily lives, for example by helping us to avoid traffic jams or suggesting better routes. However, trajectory data can also reveal sensitive information of users in the form of the addresses and places that they have visited, like hospitals, doctor's offices, or bureaus of political parties. We want to preserve the utility of shared trajectory data, while avoiding the information leak for individuals.
Use cases
- Anonymization for trajectory data
- Private learning for trajectory data
Methods
- Adaptation of existing differential privacy mechanisms
- Creation of new mechanisms specifically tailored for trajectory data
- Definitions of new privacy metrics and notions to understand when a trajectory database is actually protected
Future
- Development of new privacy-preserving mechanisms that overcome the limitations of existing mechanisms, especially considering the data correlations that exist in (trajectory) databases
- Anonymization for dynamic data
Anonymizing Humans in Motion
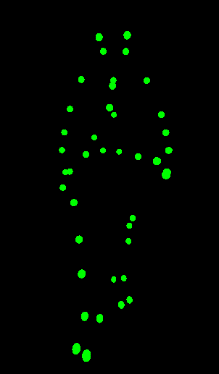
More and more data of human motion is captured, both due to the increasing technical feasibility (motion capture suits, depth cameras) as well as the increasing applications for it (such as virtual reality). As a result, there are two main questions: what makes human motion unique and what is important for the recognition of individuals? And how can we use human motion data without creating a privacy problem for the recorded person?
Use cases
- Anonymization for human motion data publishing
- Removing sensitive attributes (age, gender, …) from human motion data
Methods
- User studies to collect suitable biometric data for anonymization
- Machine learning to build recognition techniques against which we can evaluate
- Systematic feature analysis to understand the recognition process
- Anonymization technique development
Future
- Development of a better methodology for evaluating biometric anonymization performance
- Anonymization techniques for human gait
- Anonymization techniques for freehand gesture controls
Security and Privacy of Behavioral Data-Driven Applications
Nowadays, everybody has to authenticate themselves several times per day — even you :-) Most of the time, we still use passwords for that, which come with many pitfalls. Can we do better?
Use cases
- Could be used everywhere, however, our current focus is on the Industry 4.0 (the next generation of factories!)
- We want to enable easy, hands-free authentication for workers
Methods
- Use AI on behavioral data to develop novel biometric systems that are secure, usable and provide a better level of privacy
- Build on behavioral biometrics like brainwaves, eye-gaze and more
Future
- Next generation of authentication systems
Privacy-Preserving Machine Learning
A lot of modern data analysis is done via machine learning and can create huge benefits for societies and businesses alike. However, not only do these models require a lot of data during their training, including personal and/or sensitive data, the final models also can leak information about the data that was used to train them. Privacy-preserving machine learning methods exist to mitigate these emerging privacy risks, but introduce an inherent privacy-utility trade-off, inhibiting their adoption. In our research, we aim to improve the applicability of privacy-preserving machine learning by understanding and alleviating this privacy-utility trade-off.
Use cases
- Train machine learning models with privacy guarantees on sensitive data
- Machine learning based generation of syntactic data with privacy guarantees
- Privacy-preserving generation and analysis of web click traces
Methods
- Empirical studies to improve the understanding of how different parts of machine learning pipelines affect the privacy-utility trade-off and the model's resistance to attacks
- Privacy threat modelling of machine learning based systems
- Develop generative models with differential privacy guarantees
Future
- Minimize data collection through on device model training
Publications on this topic
Hanisch, S.; Arias-Cabarcos, P.; Parra-Arnau, J.; Strufe, T.
2025. ACM Computing Surveys, 57. doi:10.1145/3729418
Fallahi, M.; Arias-Cabarcos, P.; Strufe, T.
2025. CHI EA ’25: Proceedings of the Extended Abstracts of the CHI Conference on Human Factors in Computing Systems.Ed.: N. Yamashita, 1–14, Association for Computing Machinery (ACM). doi:10.1145/3706599.3720118
Pogrzeba, L.; Muschter, E.; Hanisch, S.; Wardhani, V. Y. P.; Strufe, T.; Fitzek, F. H. P.; Li, S.-C.
2025. Scientific Data, 12 (1), Art.-Nr.: 531. doi:10.1038/s41597-025-04818-y
Tamayo Gonzalez, C. C.; Soderi, S.; Todt, J.; Strufe, T.; Conti, M.
2025. 2025 IEEE Wireless Communications and Networking Conference (WCNC), 1–6, Institute of Electrical and Electronics Engineers (IEEE). doi:10.1109/WCNC61545.2025.10978264
Miranda-Pascual, À.; Guerra-Balboa, P.; Parra-Arnau, J.; Forné, J.; Strufe, T.
2024. International Journal of Information Security, 23 (6), 3711–3747. doi:10.1007/s10207-024-00894-0
Guerra-Balboa, P.; Sauer, A.; Strufe, T.
2024. WPES ’24: Proceedings of the 23rd Workshop on Privacy in the Electronic Society. Ed.: E. Ayday, 155–171, Association for Computing Machinery (ACM). doi:10.1145/3689943.3695046
Hanisch, S.; Pogrzeba, L.; Muschter, E.; Li, S.-C.; Strufe, T.
2024. Scientific Data, 11 (1), Art.-Nr.: 1209. doi:10.1038/s41597-024-04020-6
Miranda-Pascual, À.; Guerra-Balboa, P.; Parra-Arnau, J.; Strufe, T.
2024. Proceedings of the XVIII Spanish Meeting on Cryptology and Information Security (RECSI), León, 22th - 25st October, 2024
Chaurasia, A. K.; Fallahi, M.; Strufe, T.; Terhörst, P.; Cabarcos, P. A.
2024. Journal of Information Security and Applications, 85, Article no: 103832. doi:10.1016/j.jisa.2024.103832
Guerra-Balboa, P.; Miranda-Pascual, À.; Parra-Arnau, J.; Strufe, T.
2024. 2024 IEEE 37th Computer Security Foundations Symposium (CSF), 8th-12th July 2024, Institute of Electrical and Electronics Engineers (IEEE). doi:10.1109/CSF61375.2024.00004
Morsbach, F.; Reubold, J. L.; Strufe, T.
2024. 40th Annual Computer Security Applications Conference (ACSAC), Honolulu, HI, USA, 09-13 December 2024, 1217–1230, Institute of Electrical and Electronics Engineers (IEEE). doi:10.1109/ACSAC63791.2024.00097
Todt, J.; Hanisch, S.; Strufe, T.
2024. Proceedings on Privacy Enhancing Technologies, 2024 (4), 24–43. doi:10.56553/popets-2024-0105
Hanisch, S.; Todt, J.; Patino, J.; Evans, N.; Strufe, T.
2024. Proceedings on Privacy Enhancing Technologies, 2024 (1), 116–132. doi:10.56553/popets-2024-0008
Fallahi, M.; Arias-Cabarcos, P.; Strufe, T.
2023. CCS’ 23: Proceedings of the 2023 ACM SIGSAC Conference on Computer and Communications Security, Kopenhagen, 26th-30th November 2023, 3627–3629, Association for Computing Machinery (ACM). doi:10.1145/3576915.3624399
Arias-Cabarcos, P.; Fallahi, M.; Habrich, T.; Schulze, K.; Becker, C.; Strufe, T.
2023. ACM Transactions on Privacy and Security, 26 (3), Art.-Nr.: 26. doi:10.1145/3579356
Fallahi, M.; Strufe, T.; Arias-Cabarcos, P.
2023. 2023 IEEE International Conference on Pervasive Computing and Communications (PerCom), 53–60, Institute of Electrical and Electronics Engineers (IEEE). doi:10.1109/PERCOM56429.2023.10099367
Hanisch, S.; Todt, J.; Volkamer, M.; Strufe, T.
2023. Daten-Fairness in einer globalisierten Welt. Ed.: M. Friedewald, 423–444, Nomos Verlagsgesellschaft. doi:10.5771/9783748938743-423
Miranda-Pascual, À.; Guerra-Balboa, P.; Parra-Arnau, J.; Forné, J.; Strufe, T.
2023. Proceedings on Privacy Enhancing Technologies, 496–516, De Gruyter. doi:10.56553/popets-2023-0065
Hanisch, S.; Muschter, E.; Hatzipanayioti, A.; Li, S.-C.; Strufe, T.
2023. Proceedings on Privacy Enhancing Technologies (PoPETs), 177–189. doi:10.56553/popets-2023-0011
Guerra-Balboa, P.; Miranda-Pascual, À.; Parra-Arnau, J.; Forné, J.; Strufe, T.
2022. Proceedings of the XVII Spanish Meeting on Cryptology and Information Security (RECSI), Santader, 19th - 21st October, 2022. doi:10.22429/Euc2022.028
Hasebrook, N.; Morsbach, F.; Kannengießer, N.; Zöller, M.; Franke, J.; Lindauer, M.; Hutter, F.; Sunyaev, A.
2022. Karlsruher Institut für Technologie (KIT). doi:10.48550/ARXIV.2203.01717
Guerra-Balboa, P.; Miranda-Pascual, À.; Strufe, T.; Parra-Arnau, J.; Forné, J.
2022. AAAI Workshop on Privacy-Preserving Artificial Intelligence
Rettlinger, S.; Knaus, B.; Wieczorek, F.; Ivakko, N.; Hanisch, S.; Nguyen, G. T.; Strufe, T.; Fitzek, F. H. P.
2022. 2022 IEEE International Conference on Pervasive Computing and Communications Workshops and other Affiliated Events (PerCom Workshops), Pisa, Italy, 21-25 March 2022, 88–90, Institute of Electrical and Electronics Engineers (IEEE). doi:10.1109/PerComWorkshops53856.2022.9767484
Morsbach, F.; Dehling, T.; Sunyaev, A.
2021. Presented at NeurIPS 2021 Workshop on Privacy in Machine Learning (PriML 2021), 14.12.2021. doi:10.48550/arXiv.2111.14924
Boenisch, F.; Munz, R.; Tiepelt, M.; Hanisch, S.; Kuhn, C.; Francis, P.
2021. CCS ’21: Proceedings of the 2021 ACM SIGSAC Conference on Computer and Communications Security, November 2021, 1254–1265, Association for Computing Machinery (ACM). doi:10.1145/3460120.3484751
Hanisch, S.; Arias-Cabarcos, P.; Parra-Arnau, J.; Strufe, T.
2021. arxiv. doi:10.5445/IR/1000139989
Aßmann, U.; Baier, C.; Dubslaff, C.; Grzelak, D.; Hanisch, S.; Hartono, A. P. P.; Köpsell, S.; Lin, T.; Strufe, T.
2021. Tactile Internet. Ed.: F. H.P. Fitzek, 293–317, Academic Press. doi:10.1016/B978-0-12-821343-8.00025-3